Identifying Key Collective Variables in High-Dimensional MD Simulations
Machine learned CVs for QM/MM enhanced sampling simulations
Tackling the challenges of identifying CVs, machine learning (ML) approaches can help to effectively map noisy high dimensional spaces to the minimal essential space. To this end, we aim to explore uncharted territory in developing and testing strategies to augment QM/MM simulations by machine learned CVs. A case in point is our studies on disulfide shuffling in protein systems. This reaction is highly dynamic and sensitive to the electrostatic environment. This hampers state exploration and can obscure environmental effects due to insufficient sampling. With pertinent CVs we can improve sampling of environmental effects and gain susceptibility to mechanistic details hidden within a vast amount of MD data.
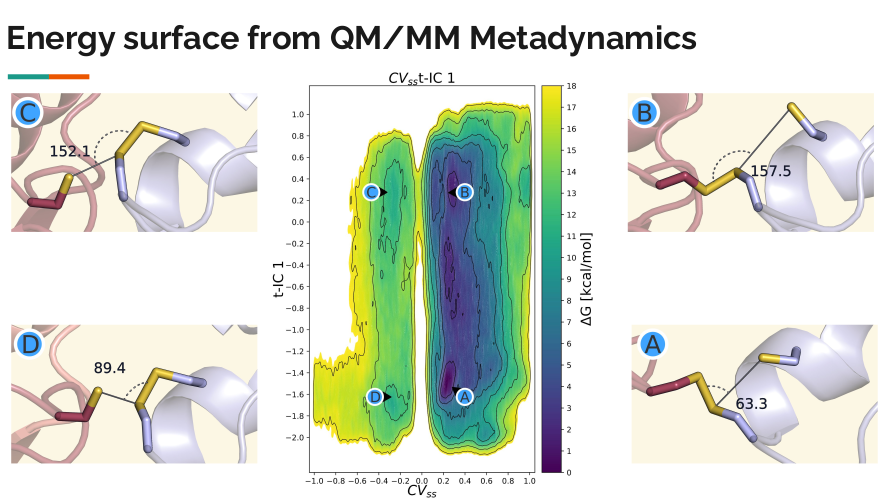
of disulfide shuffling, as introduced below, our QM/MM enhanced sampling approach employs
asymmetric distance restraints to drive the chemical reaction. This is combined with a collective
variable (CV) derived from time-lagged independent component analysis (tICA), which captures
conformational changes in the disulfide bridge. Through this approach, we characterized the struc-
tural clusters of conformations that the system traverses to achieve the optimal transition state
geometry. Furthermore, we have investigated how the interplay between reactivity and dynamics
of the system is affected.
Understanding Kinase Reactions through QM/MM and Metadynamics
Simulations
Kinases are crucial enzymes that catalyze the transfer of phosphate groups and play critical roles in energy metabolism, signal transduction, and cellular regulation. Our research aims to understand their complex mechanisms at the atomic level in order to gain deeper insight into their functions. To achieve this, we employ a quantum mechanics/molecular mechanics (QM/MM) approach, where molecular mechanics (MM) describes the protein environment, while density functional tight-binding (DFTB) provides a more detailed representation of the active site. Enhanced sampling techniques, such as metadynamics, accelerate the exploration of reaction pathways and
reveal the free energy surface. This allows us to trace reaction mechanisms, quantify thermodynamic and kinetic properties, and validate our findings through comparison with experimental data.
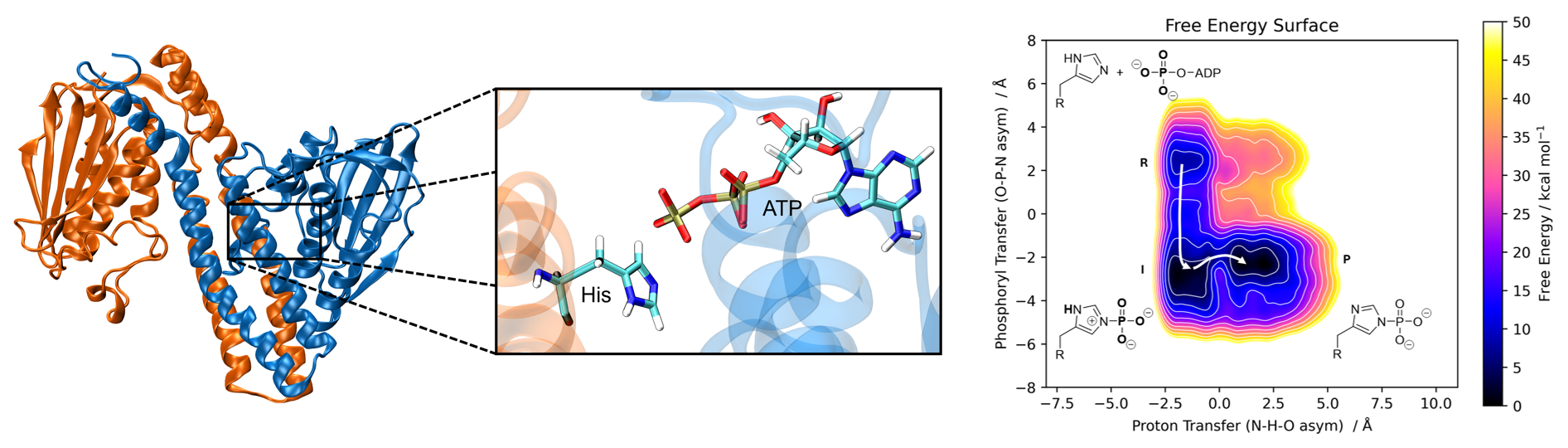
of ATP gets transferred onto a conserved histidine residue. Right: Free energy surface of the
phosphoryl transfer reaction from ATP to histidine with a subsequent proton transfer to a nearby
base.
Master’s Thesis: QM/MM Analysis of Glutathionylation Reaction Energy in PfGrx Wildtype and Active Site Mutants
Glutaredoxins (Grx) are enzymes involved in cellular redox homeostasis, regulating thiol- disulfide exchange (TDE) reactions to maintain protein function under oxidative stress. This thesis investigates the interaction of Grx with glutathione disulfide (GSSG) and the role of active site mutations (Y37A, Y37F and K32E) in modulating its activity. A combi- nation of different computational methods, including alchemical transitions, well-tempered metadynamics (WTMetaD), and quantum mechanics/molecular mechanics (QM/MM) simulations, are used to evaluate the relative binding free energies of GSSG to wild type Grx and its reaction dynamics.
Key findings include the identification of the aromtatic ring of the tyrosine residue at position 37 in the CPYC active site motif as critical for GSSG binding, while the positively charged lysine residue at position 32 stabilizes the negatively charged thiolate in the active site through attractive electrostatic interactions. These results align with prior experimental studies and highlight the importance of the active site residues in modulating Grx activity.
The study also addresses methodological challenges encountered during the WTMetaD simulations, particularly the difficulty in assessing the convergence of the resulting free energy surface (FES). The development and application of block averaging for the analysis of the current WTMetaD setup provides a systematic approach to evaluate convergence, establishing a reliable protocol for refining metadynamics setups. Overall, this work advances the understanding of Grx catalysis, offering insights into key residues involved in substrate binding. It also provides a robust computational framework for future refinements of the WTMetaD setup, enabling deeper exploration of the activity of Grx regarding the TDE reaction and its implication in redox biology.
Master Thesis: Identifying Reaction Coordinates for Complex Transitions in Proteins: Application to Histidine Kinase
Complex conformational transitions are essential for the gating of enzymes between distinct functional states. For instance, the protein histidine kinase (HK) transduces extracellular signals through a series of conformational changes that ultimately lead to an autophosphorylation reaction at the catalytic core. The actual transition is a rare event, occurring on millisecond timescales. These extended sampling times present a significant computational challenge. For this reason it is advantageous to use enhanced sampling methods to accelerate the movement along predefined reaction coordinates. For this reason it is advantageous to use enhanced sampling methods to accelerate the movement along predefined reaction coordinates. However, the selection of suitable
reaction coordinates is challenging, especially for high-dimensional and complex motions in large biomolecular systems. Until now, coordinates for large systems such as the HK have been determined on the basis of chemical and geometric intuition. In this study an objective and generic approach was developed.
ML-based methods such as Principal Component Analysis (PCA), Autoencoders, or Time-lagged Independent Component Analysis (TICA) aim to reduce the dimensionality of the system while preserving its essential dynamics. These methods analyze simulation data to identify collective motions or patterns that drive the transition between states. In doing so, they allow the construction of effective reaction coordinates, facilitating enhanced sampling methods such as metadynamics. This approach allows the study of conformational transitions, the determination of transition states, and the estimation of activation barriers, ultimately providing a deeper understanding of the underlying mechanisms of biochemical processes.